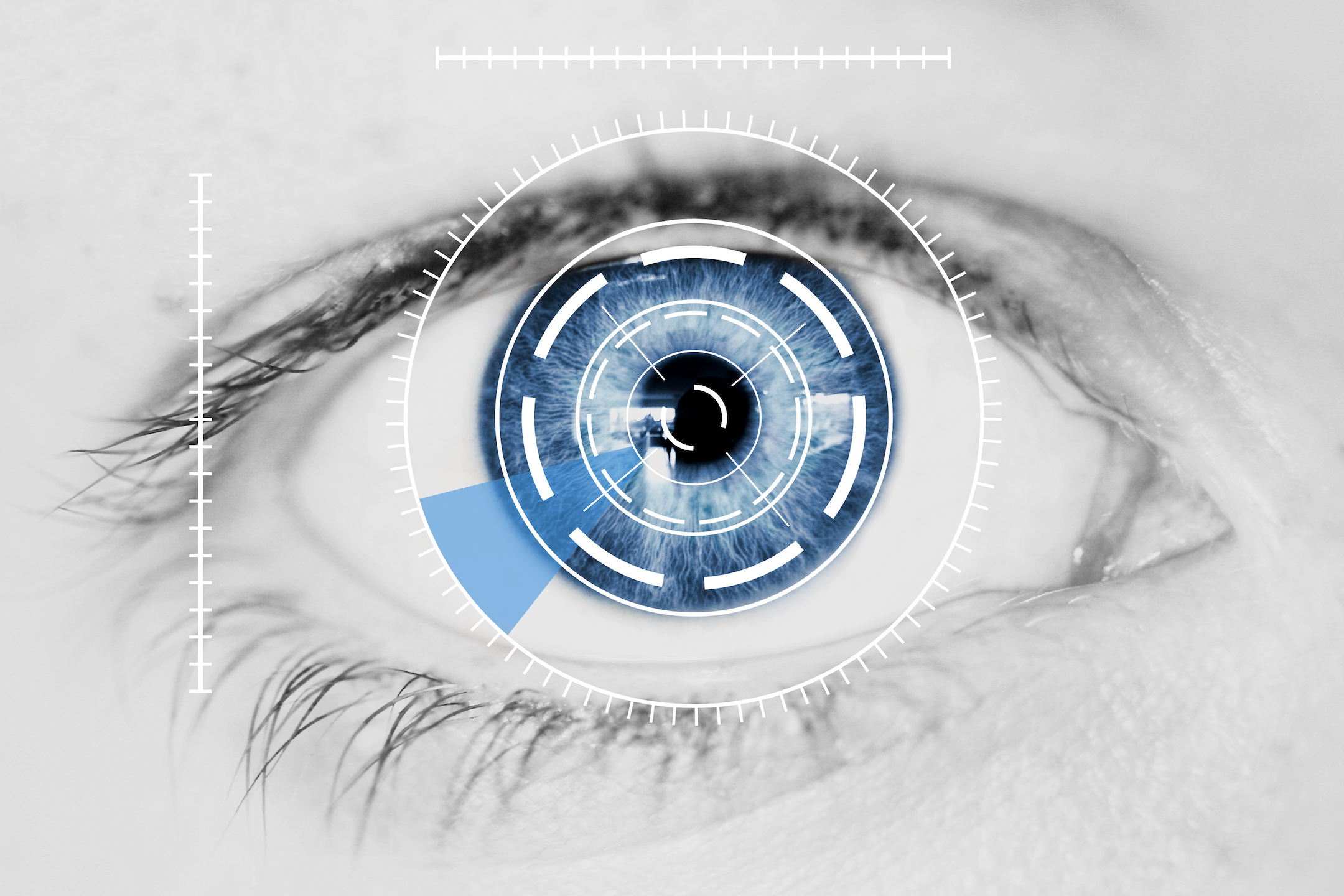
Machine-learning Assisted Detection of Age-related Macular Degeneration from Fundus Photos
Researchers proposed a model for the early detection of AMD using various ML architectures. Their model ranked fourth in the ADAM challenge and was found to be extremely lightweight while being comparable in performance to other state-of-the-art models.
NCKU researchers propose various machine-learning architectures to solve the four tasks in the ADAM challenge
Age-related macular degeneration (AMD) causes vision loss in one or both eyes when left untreated. Early detection is, therefore, essential to prevent permanent damage to the eyes. However, diagnosing it via fundus images is time-consuming and requires expert input from doctors. To solve these issues, the ADAM challenge was set up for machine learning-assisted early detection of AMD. Out of the 11 participating teams, the team from NCKU, Taiwan, had the fourth-best ranking model.
Vision loss is common among the elderly, and age-related macular degeneration (AMD) is one of the leading causes of vision loss. The macula is a region located in the retina and AMD causes lesions to appear on the macula, leading to fuzzy or distorted vision and central vision loss in one or both eyes. According to clinical characterization, AMD can be divided into three stages—early, intermediate, and late. And, if left untreated, AMD can lead to permanent vision loss and irreversible damage to the retina.
The recent rise of machine learning (ML) in medical applications promises a quick and powerful solution for the early detection of AMD, which is essential for timely treatment. Fundus photography is a powerful tool that can be used to detect AMD; however, it is time-consuming and requires the expertise of a medical professional.
To this end, the Automatic Detection challenge on Age-related Macular degeneration or the ADAM challenge was set up as a satellite event of the ISBI 2020 conference. Eleven teams from all over the world participated in the challenge. The details of the challenge and its results were made available online in IEEE Transactions on Medical Imaging on May 4, 2022, and subsequently published in Volume 41 of the journal in October 2022. The team from National Cheng Kung University (NCKU), led by Dr. Chih-Chung Hsu, an Assistant Professor at the Institute of Data Science, NCKU, proposed the fourth-best ranking model for the ADAM challenge.
The challenge was broken down into four tasks addressing the critical aspects of detecting and characterizing AMD—AMD classification, optic disc detection and segmentation, fovea localization, and lesion detection and segmentation. A dataset of 1200 fundus images was made available for the challenge. The team from NCKU used four different ML architectures to address each task of the ADAM challenge.
For the first task, the team used an EfficientNet architecture for the binary classification of AMD. Then, for the optic disc detection and segmentation task, they used the EfficientNet architecture for classification combined with U-Net for segmentation with a weight cross-entropy loss function. For the fovea localization task, the team used a combination of two U-Net architectures, a Mask-RCNN and ResNet. And for the last task of lesion detection and segmentation, they used a DeepLab-v3 with Resnet.
Out of the four architectures used, the one used for fovea localization particularly stood out. The team used a multiple-blocks regression strategy to divide the fundus image into multiple blocks, after which each block was one-hot-encoded. “Early detection of AMD is essential for its treatment. We wanted to achieve a highly accurate symptom localization for fundus image, helping the doctors to have external references for their diagnosis,” says Dr. Hsu about the ML architecture.
The model proposed by the NCKU team was comparable in performance to other state-of-the-art models while being extremely lightweight. Additionally, the ensemble strategy employed improved the performance of the model and the use of prior clinical knowledge helped to achieve better results. “Given the fundus image, it is possible to diagnose AMD in a real-time sense, which might reduce the effort of doctors. Further, the proposed model can be integrated into hardware to be one of the standard pieces of equipment in any clinic. That would provide us with an AI-aided diagnosis, which could be very helpful for junior doctors or even interns,” concludes Dr. Hsu.
Here’s looking forward to seeing this model in use in standardized equipment across hospitals and clinics soon!
Reference
AuthorsHuihui Fang1, Fei Li2, Huazhu Fu3, Xu Sun1, Xingxing Cao1, Fengbin Lin2, Jaemin Son6, Sunho Kim6, Gwenole Quellec7, Sarah Matta7, Sharath M Shankaranarayana8, Yi-Ting Chen9, Chuen-heng Wang10, Nisarg A. Shah11, Chia-Yen Lee12, Chih-Chung Hsu13, Hai Xie14, Baiying Lei14, Ujjwal Baid15, Shubham Innani15, Kang Dang16, Wenxiu Shi16, Ravi Kamble17, Nitin Singhal17, Ching-Wei Wang18, Shih-Chang Lo18, José Ignacio Orlando4, Hrvoje Bogunović5, Xiulan Zhang2,*, Yanwu Xu1,*, iChallenge-AMD study group
ADAM Challenge: Detecting Age-Related Macular Degeneration From Fundus Images
IEEE Transactions on Medical Imaging
Affiliations
1Intelligent Healthcare Unit, Baidu Inc., China
2Sun Yat-sen University, China
3Agency for Science, Technology and Research, Singapore
4Yatiris lab of Pladema Institute, Argentina
5Medical University of Vienna, Austria
6VUNO Inc. Seoul, Republic of Korea
7Inserm, France
8Zasti India Pvt. Ltd, India
9Graphen.Inc., Taiwan
10Muen Biomedical and Optoelectronics Technologies Inc., Taiwan
11Indian Institute of Technology, Rajasthan, India
12National United University, Taiwan
13National Cheng Kung University, Taiwan
14Shenzhen University, China
15SGGS Institute of Engineering and Technology, India
16Suzhou Tisu Information Technology Co. Ltd., China
17AIRA Matrix, India
18National Taiwan University of Science and Technology, Taiwan
Key Academics
About Professor Chih-Chung HsuChih-Chung Hsu is an Assistant Professor at the Institute of Data Science at NCKU, Taiwan. He is also an IEEE Senior Member. He received his Ph.D. in Electrical Engineering from National Tsing Hua University in Taiwan. His research interests are in the fields of image processing, computer vision, deep learning, and machine learning. In the past, he has worked in the industry as a co-founder of SKOPY Inc. and Eye-Digit. Inc. He has 66 articles credited to him and 1176 citations to his name.